Artificial intelligence (AI) has brought many advances in different fields, such as speech recognition, image understanding, robotics, and autonomous vehicles, tackling problems for which the general belief was that only human intelligence could solve. The chess-playing computer dubbed Deep Blue, the question-answering computer dubbed Watson, and recently self-driving cars are well-known examples of different AI-machines performing human tasks. The capability to “understand” their surroundings via digital cameras, or in other words, to understand images is one of the main requirements for self-driving cars. In this post we showcase how this seemingly magical aspect of AI can be used in pharmaceutical inspection technology for quality assurance.
QUALITY ASSURANCE
The main goal of pharmaceutical inspection technology is quality assurance of the end product, achieved via the meticulous inspection of every single product. Nowadays, a variety of different pharmaceutical product types are available, ranging from different sizes, shapes, and colors to different opacities as illustrated in Figure 1. Visual inspection of these products is an important and regulated task, whereby the inspection process aims to avoid defective or substandard pharmaceutical products by detecting various defects that arise during the production process. The occurring defects range from critical, which affect the product’s functional aspect such as cracks, dents, scratches, and foreign particles to defects that merely affect the visual appearance of the product such as impurities and smudges. Automated visual inspection addresses the inspection requirements of large quantities and the ever-growing variety of pharmaceutical products, by means of specialized machines and engineered defect-detection solutions. For some visual inspection problems, however, engineering complex solutions can sometimes prove to be a very hard or an altogether impossible task. Visual inspection of translucent objects, such as transparent capsules and softgels, falls into this category. Due to their inherent optical properties, which affect the overall visual complexity, these are mostly inspected manually by a human operator.
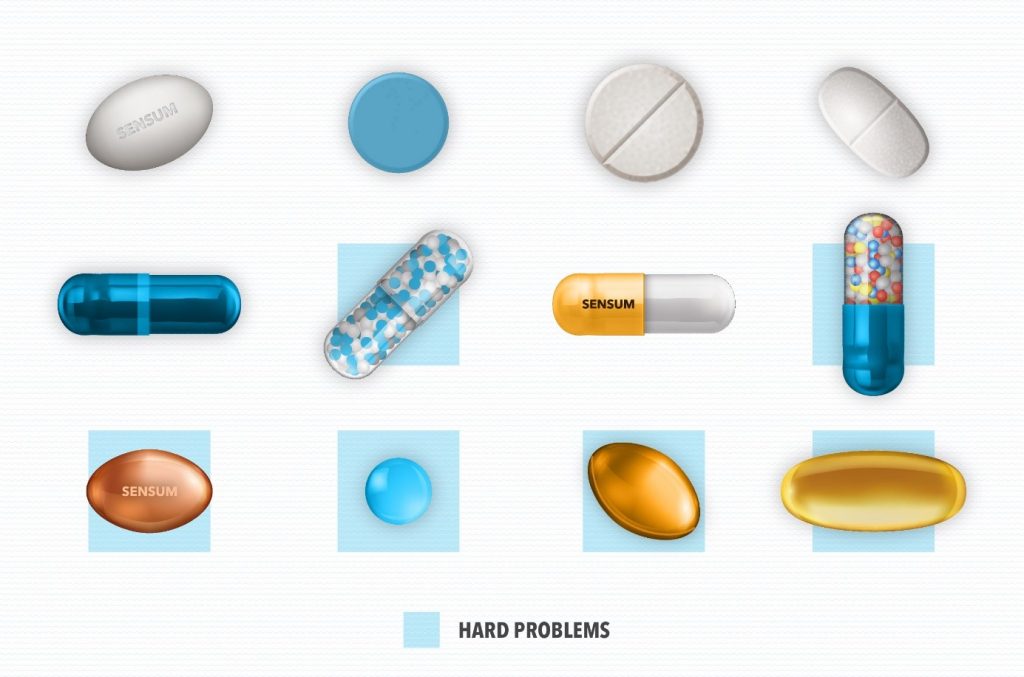
Figure 1: Pharmaceutical product types of different sizes, shapes, colors, and different opacities.
UNDERSTANDING IMAGES
Technological advancement, i.e., the development of new or the improvement of existing solutions is achieved via the process of critical analysis of emerging technologies. AI’s magic hat contains a lot of different tricks, the most magical trick of all allows an AI-machine to learn-to-understand images and is called Deep Learning (DL). Applied in the right context, DL has proven to perform better than existing approaches in solving very complex problems. Let’s reveal some of the magic behind this seemingly mystifying trick of AI, and take a look at how a DL-based solution in the pharmaceutical inspection domain can be obtained in three critical steps, i.e., by specifying the inspection problem with physical product samples; learning how to separate these samples; and integrating the obtained solution into an inspection machine.
1 PROBLEM SPECIFICATION – GARBAGE IN, GARBAGE OUT
There is a well-known saying in the scientific field of Machine Learning, i.e., “garbage in, garbage out”, and it refers to the fact that if a problem is poorly specified, the resulting problem solution will be of poor quality. AI’s magic trick won’t work on its own – nothing really works on its own.
In order to obtain reasonable solutions to inspection and quality control problems, the first critical step is to involve the pharmaceutical product manufacturer and answer the question “what is good and what is bad?”. The answer is obtained by manually examining and physically separating samples from a product batch, consisting of both defect-free (good) and defective (bad) samples. During this process, the inspection domain experts in cooperation with the pharmaceutical product manufacturer aim to determine defects which commonly occur during the production process or at a certain stage of the production process. The appropriate specification of acceptable and nonacceptable deviations from the expected final product represents the basis, from which a solution is developed that is able to meet inspection and quality control requirements.
2 LEARNING TO SEPARATE – THE BAD FROM THE GOOD
The question “what is good and what is bad?” is answered, by humans, using their eyes, their knowledge and experience, and their hands to physically separate the product samples. How can now this knowledge, of “how to separate”, be explained to a machine? Here’s where AI’s magic learning trick comes into action.
After the problem is specified and the sample product batch is divided into good (defect-free or acceptable) and bad (defective or nonacceptable) samples, inspection domain experts capture images of product samples via digital cameras using a state-of-the-art inspection machine. The collected sample images are furthermore inspected and all visible defects are marked. At this point, a DL-based approach is utilized, which looks at every single captured image and “learns” the separation of good and bad samples. The learning phase itself involves repeated mathematical and statistical operations for the gradual construction of a complex hyperspace, via which the sample separation is achieved. Figure 2 illustrates the similarities and differences between a human and a DL-based approach to a separation task. However, since this approach alone may not always yield the required performance, further solution engineering and optimization is undertaken whereby the more problematic samples and potential failure cases are furthermore analyzed. Together, these steps ensure the development of a solution that is able to address the specified inspection problem.
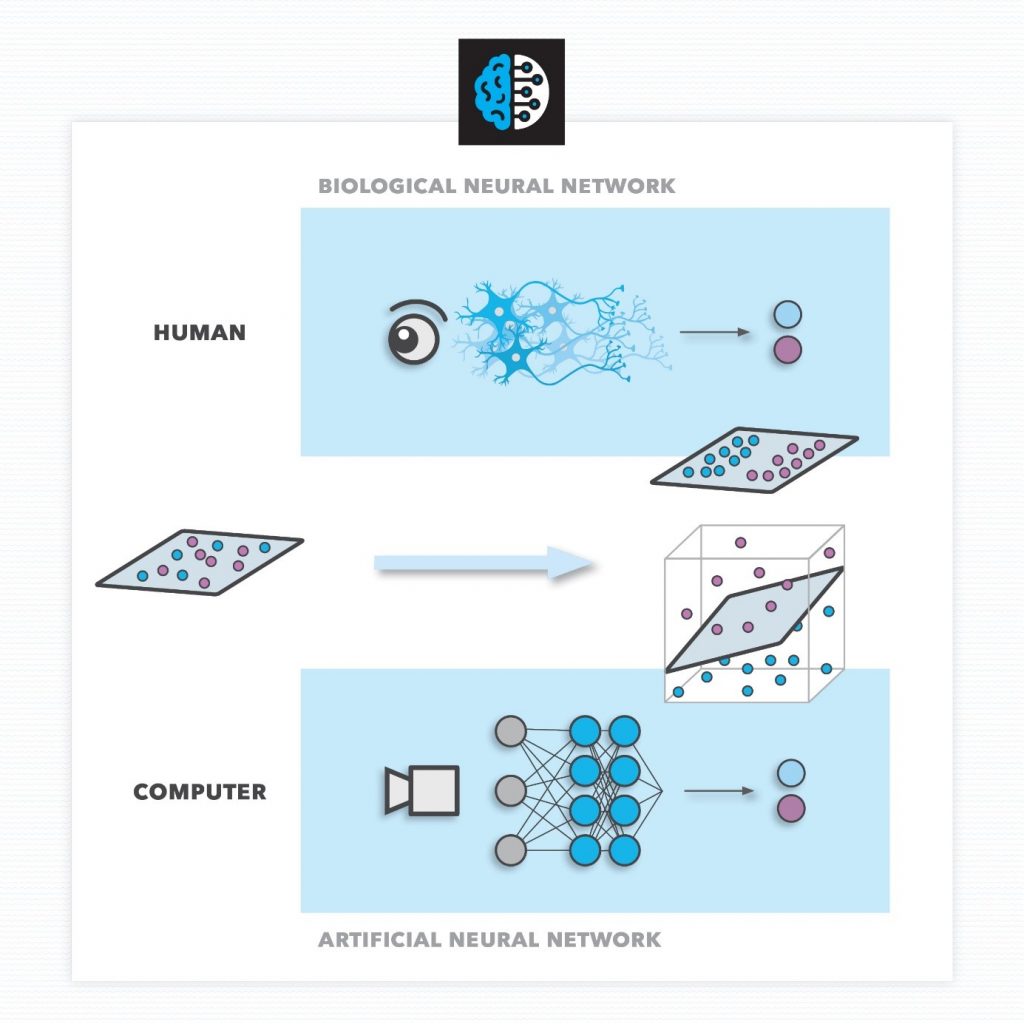
Figure 2: Similarities and differences between a human and a Deep Learning-based approach to a separation task.
3 SOLUTION INTEGRATION – THE AI-MACHINE
At this point the specific knowledge of “how to separate” has been learned from the divided physical samples, by using an AI approach in order to obtain a solution to a separation problem. The remaining step is to fuse this knowledge with an inspection machine, yielding the AI-machine.
The developed solution undergoes rigorous testing and only after successful validation is integrated into a state-of-the-art inspection machine for pharmaceutical products. These machines incorporate fast mechanical manipulation, controlled illumination, fast image acquisition and analysis, and reliable defect detection and sorting mechanisms. With specialized defect-detection solutions, these highly efficient inspection systems aid the production process by performing tireless quality control on every single product, sorting out substandard products, and ensuring a reliable grade of quality for the end product.
HUMAN VS AI-MACHINE
A state-of-the-art AI-machine mimics a human by replacing the eyes with digital cameras, the hands with mechanical manipulators, and the specific knowledge with AI. In addition to faster performance, these fully automated solutions provide a higher degree of reliability and objectivity compared to human-error prone manual inspection, which becomes more and more apparent at longer operational runs. How precise and unbiased a machine vision system can be when compared to a human in visual quality control is well explained and tested in the following post.
Automated visual inspection addresses inspection requirements of large quantities, while AI provides the ability to address challenging inspection problems of the ever-growing variety of pharmaceutical products, some of which were beforehand addressed only via manual inspection. At Sensum, we develop and manufacture state-of-the-art automated machines for visual inspection, a process that has been identified as one of the most labor-intensive in the pharmaceutical production of softgels and solid dosage forms. Sensum machines help pharmaceutical companies achieve operational autonomy and allow operators to focus on higher added-value tasks. Additionally, due to their inherent non-invasiveness, they help to reduce unnecessary human interaction, which can result in significant health benefits, as explained in the following post.
Ultimately, these systems and solutions are designed to support pharmaceutical manufacturers in the quality assurance of the end product, achieved via the meticulous inspection of every single product. And even if some of the tricks for solving hard inspection problems may seem magical at first glance, let’s not forget that the magical hat of AI, which holds the magic tricks, was crafted by a human.
This article was originally published by Domen Rački, in one of his articles on LinkedIn:
https://www.linkedin.com/pulse/artificial-intelligence-pharmaceutical-inspection-technology